Paper Review: Lumiere: A Space-Time Diffusion Model for Video Generation
Lumiere is a novel text-to-video diffusion model that stands out for its ability to synthesize videos with realistic, diverse, and coherent motion. It differs from traditional models by using a Space-Time U-Net architecture, generating videos in a single pass instead of the usual method of creating keyframes and then adding details. This approach, which includes spatial and temporal down- and up-sampling, helps maintain global temporal consistency. Lumiere uses a pre-trained text-to-image diffusion model, enabling it to produce full-frame-rate, low-resolution videos at multiple space-time scales. It achieves state-of-the-art results in text-to-video generation and is adaptable for various content creation and video editing tasks, such as image-to-video conversion, video inpainting, and stylized video generation.
The approach
Lumiere uses Diffusion Probabilistic Models for video generation, which approximate a data distribution through denoising steps, starting from Gaussian noise and gradually refining it. Lumiere’s framework includes a base model for generating low-resolution video clips and a spatial super-resolution model for upscaling to high resolution.
The Space-Time U-Net (STUnet) in Lumiere downsamples both spatially and temporally, focusing computation on a compact space-time representation. This architecture, inspired by biomedical data processing techniques, interleaves temporal blocks with spatial resizing modules and incorporates temporal convolutions and attention. The temporal attention is used at the coarsest resolution to manage computational demands.
For spatial super-resolution, Lumiere uses Multidiffusion to handle memory constraints and avoid temporal artifacts. This involves splitting the video into overlapping segments, processing each with SSR, and then combining them. The combination is optimized to minimize differences between the segments and their SSR predictions, ensuring smooth transitions in the final high-resolution video.
Applications
Stylized Generation
Lumiere uses a technique inspired by GAN-based interpolation, blending the fine-tuned T2I weights with the original weights. Different styles result in distinct motion characteristics in the generated videos, with examples including watercolor painting for realistic motion and line drawing or cartoon styles for unique, non-realistic motion.
Conditional Generation
Lumiere is extended to generate videos based on additional input signals: like a noisy video, a conditioning video (or image) or a binary mask. The applications include Image-to-Video, inpainting and cinemagraphs.
Evaluations
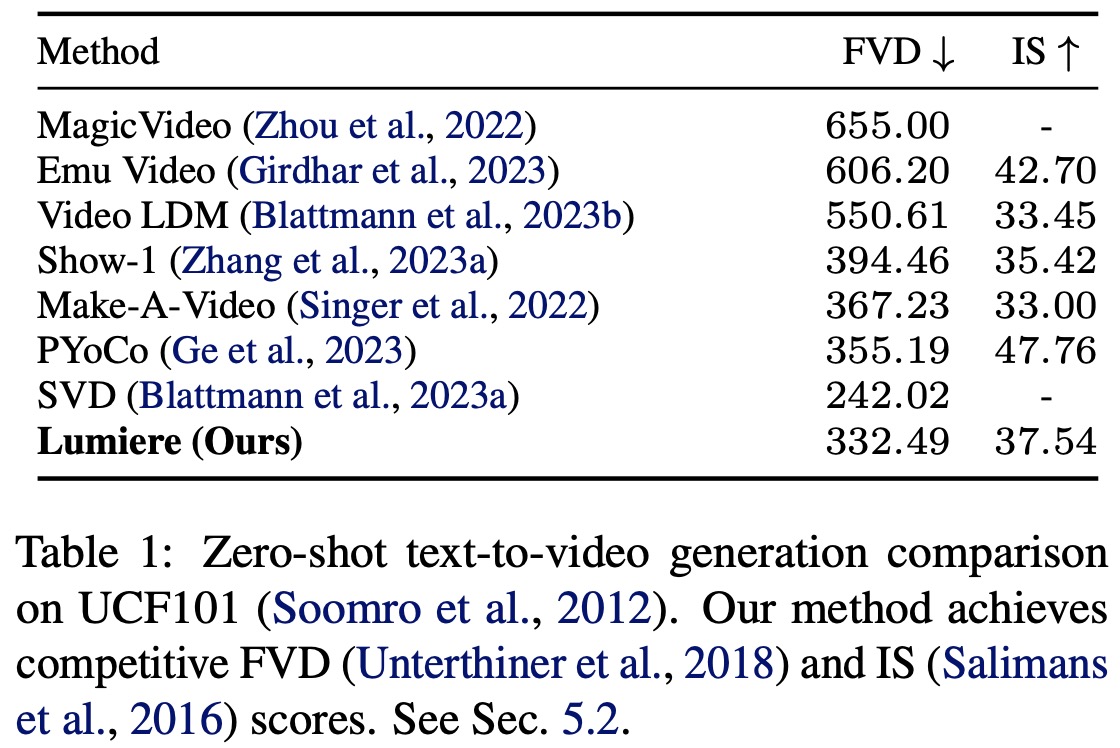
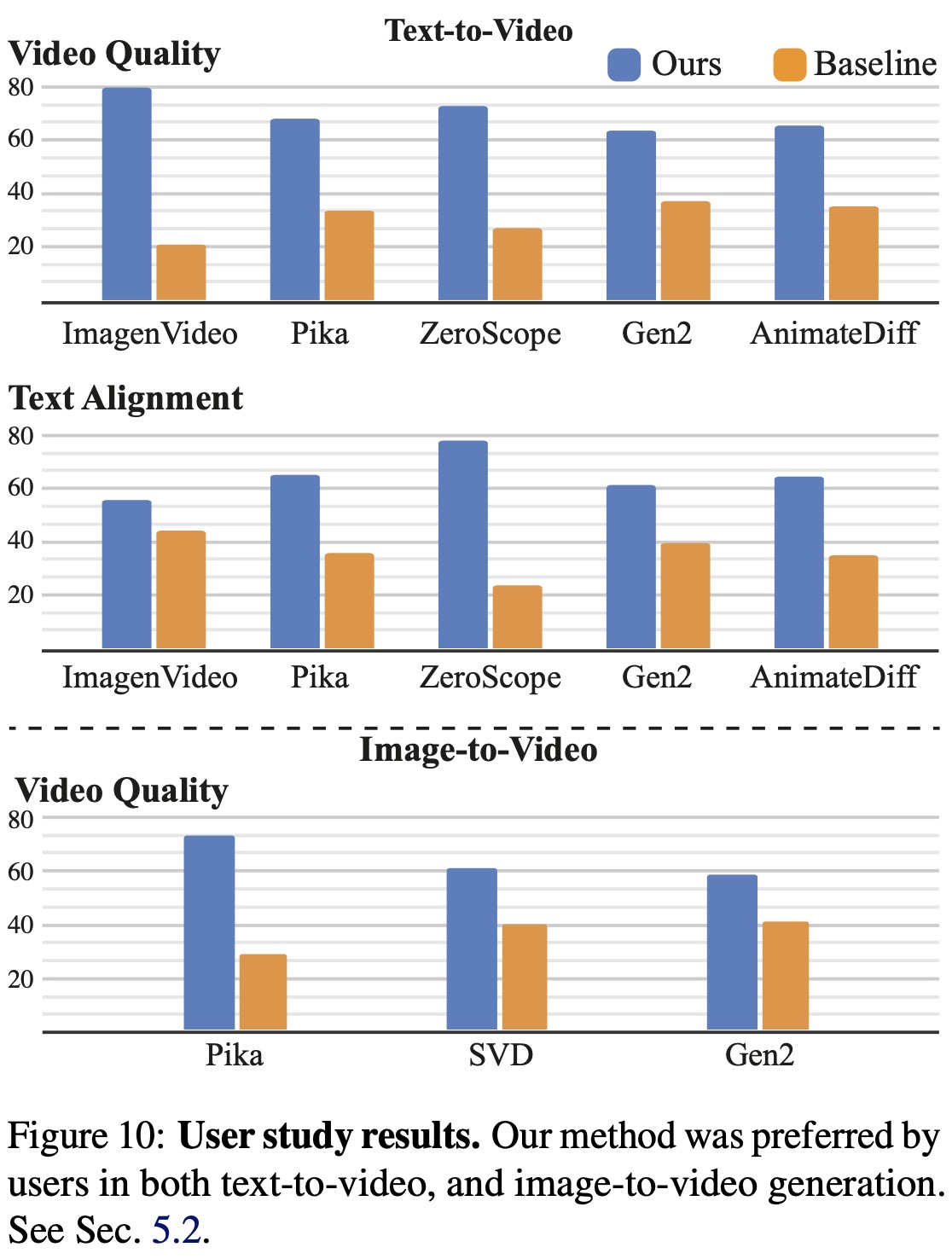
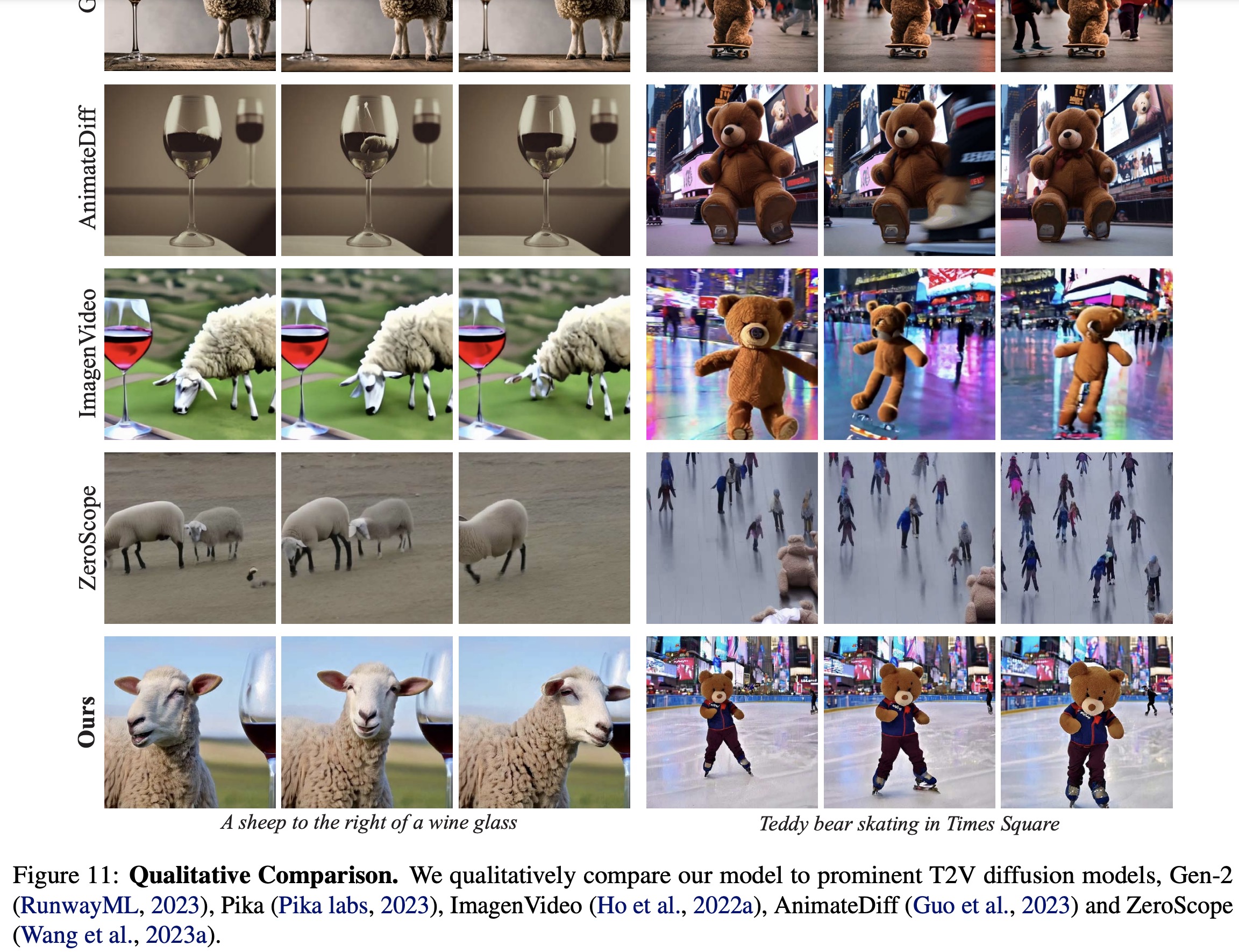
In the qualitative assessment, Lumiere outshines its competitors by producing 5-second videos with greater motion magnitude, better temporal consistency, and overall quality. Gen2 and Pika, while high in per-frame visual quality, tend to produce nearly static videos. ImagenVideo, AnimateDiff, and ZeroScope, despite showing noticeable motion, suffer from visual artifacts and generate shorter videos.
In quantitative terms, Lumiere is evaluated on the UCF101 dataset for zero-shot text-to-video generation and achieves competitive Frechet Video Distance and Inception Score metrics. However, these metrics have their limitations in accurately reflecting human perception and capturing long-term motion.
Further validation comes from a user study conducted using the Two-alternative Forced Choice protocol on Amazon Mechanical Turk. Participants compared Lumiere’s videos with those from baseline methods, focusing on visual quality, motion, and alignment with text prompts. Lumiere was consistently preferred over the baselines, indicating its superior performance in aligning with text prompts and in overall video quality.
paperreview deeplearning cv sd imagegeneration