Paper Review: STAR: Spatial-Temporal Augmentation with Text-to-Video Models for Real-World Video Super-Resolution
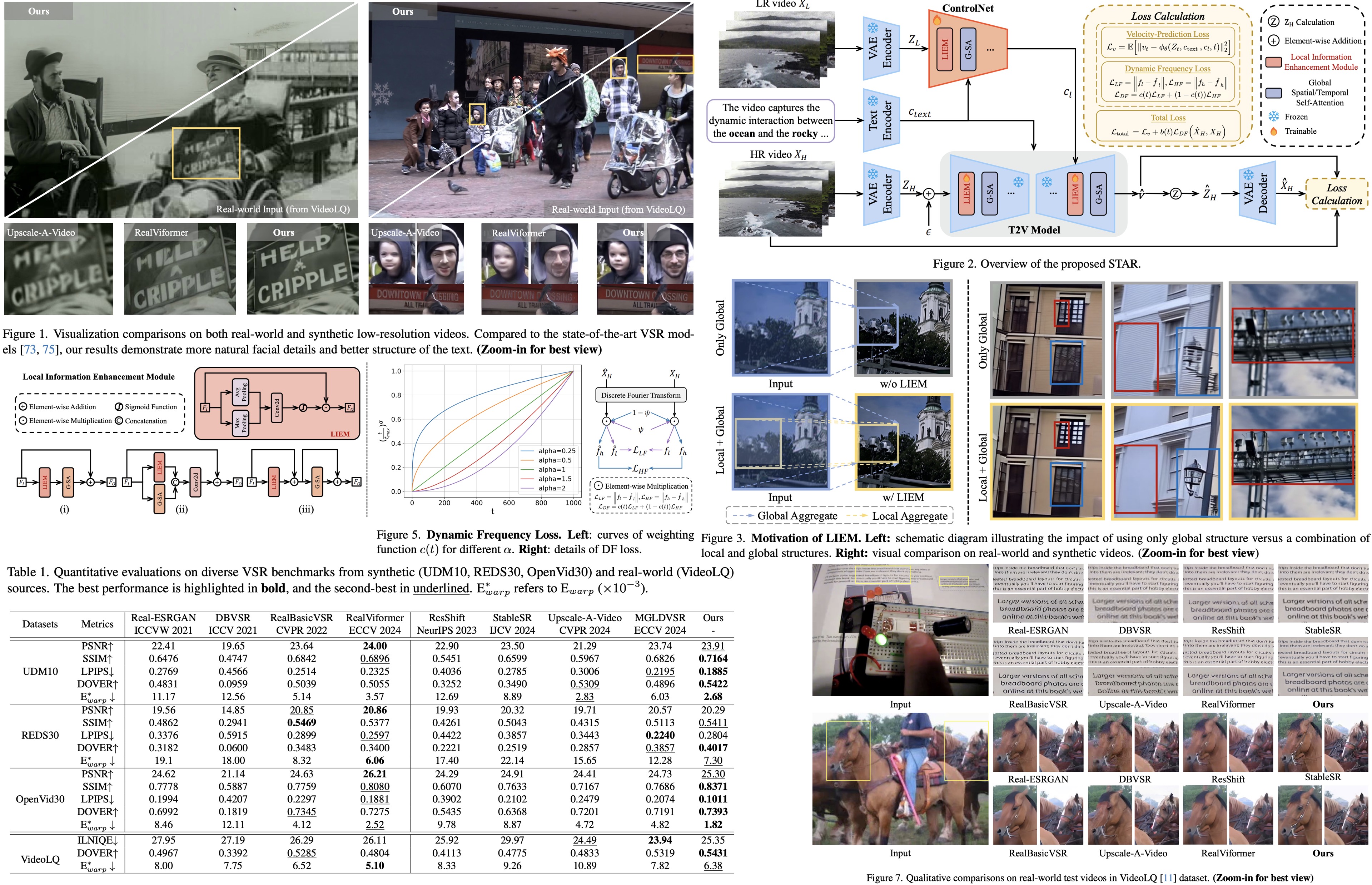
STAR improves real-world video super-resolution by addressing over-smoothing and temporal inconsistency issues in existing models. It uses T2V models for better temporal modeling while tackling artifacts from complex degradations and fidelity loss caused by strong generative capacities. STAR introduces a Local Information Enhancement Module (LIEM) to enrich spatial details and reduce artifacts and a Dynamic Frequency (DF) Loss to improve fidelity by focusing on frequency components during diffusion. It outperforms state-of-the-art methods on synthetic and real-world datasets.
The approach
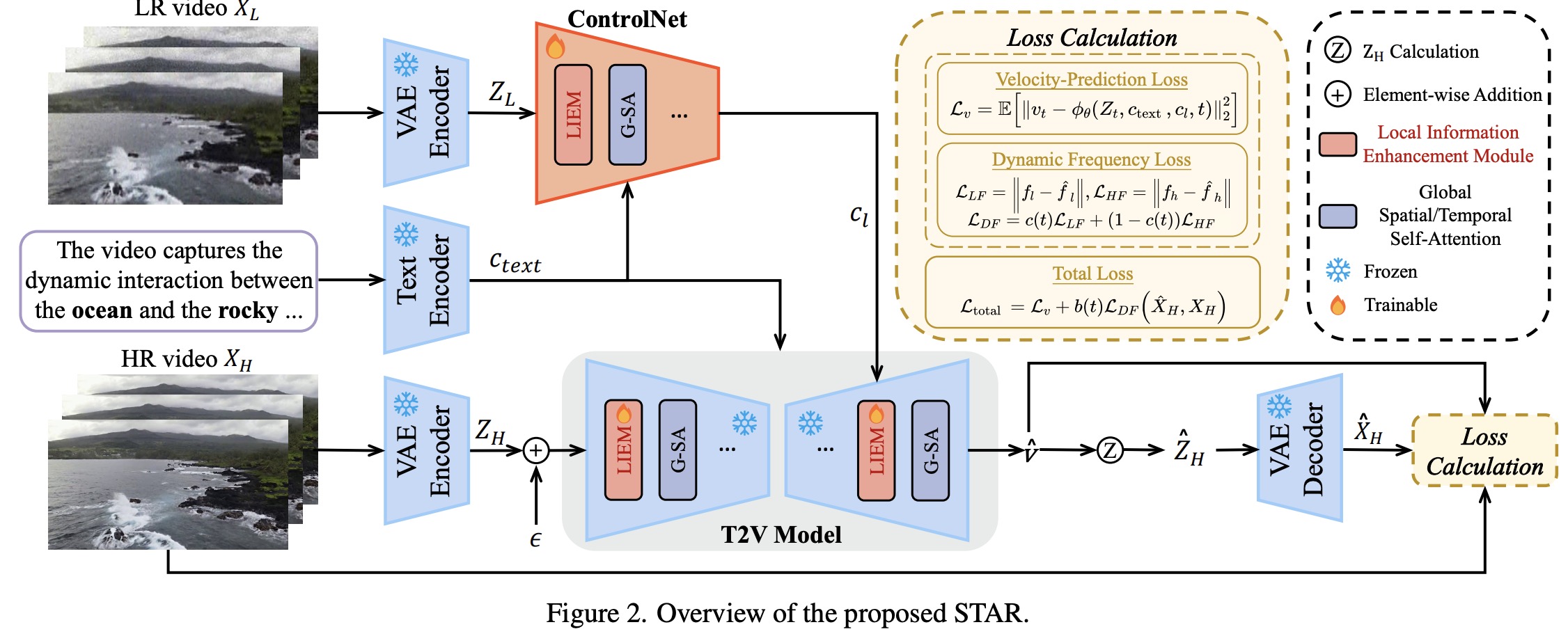
STAR consists of four main components: a VAE, a text encoder, ControlNet, and a T2V model enhanced with LIEM to reduce artifacts. The VAE generates latent tensors from high-resolution and low-resolution videos. The text encoder provides text embeddings for high-level information, which, along with latent tensors, guide the T2V model via ControlNet. The T2V model predicts velocity during diffusion steps to restore video quality.
The optimization uses a v-prediction objective to minimize velocity prediction error. To improve fidelity, STAR introduces Dynamic Frequency Loss, which dynamically adjusts constraints on high- and low-frequency components during diffusion steps. The overall loss function combines the v-prediction objective and DF Loss with a time-dependent weighting factor.
Local Information Enhancement Module
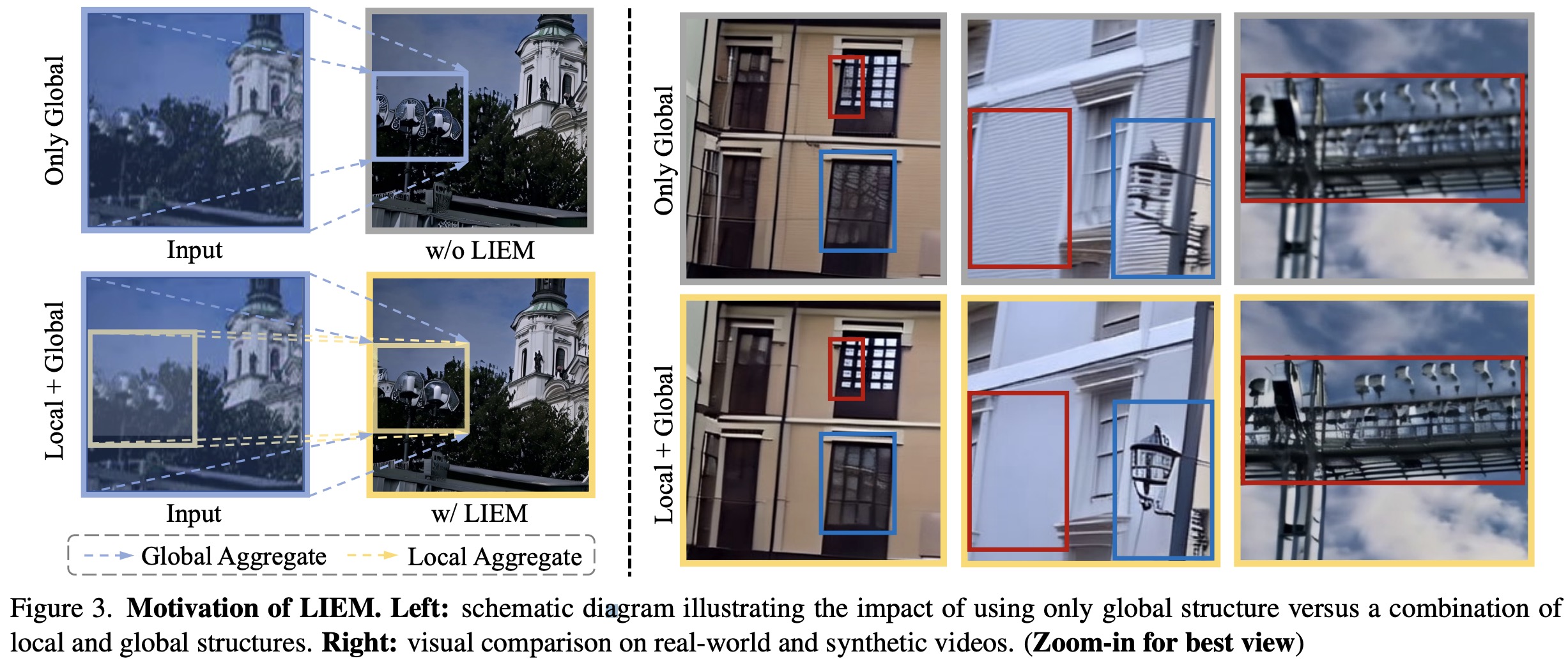
T2V models primarily rely on a global attention mechanism, which excels in generating videos from scratch but is less effective for real-world video super-resolution. They struggle with complex degradations and fail to capture local details, resulting in blurry outputs and difficulty removing artifacts.
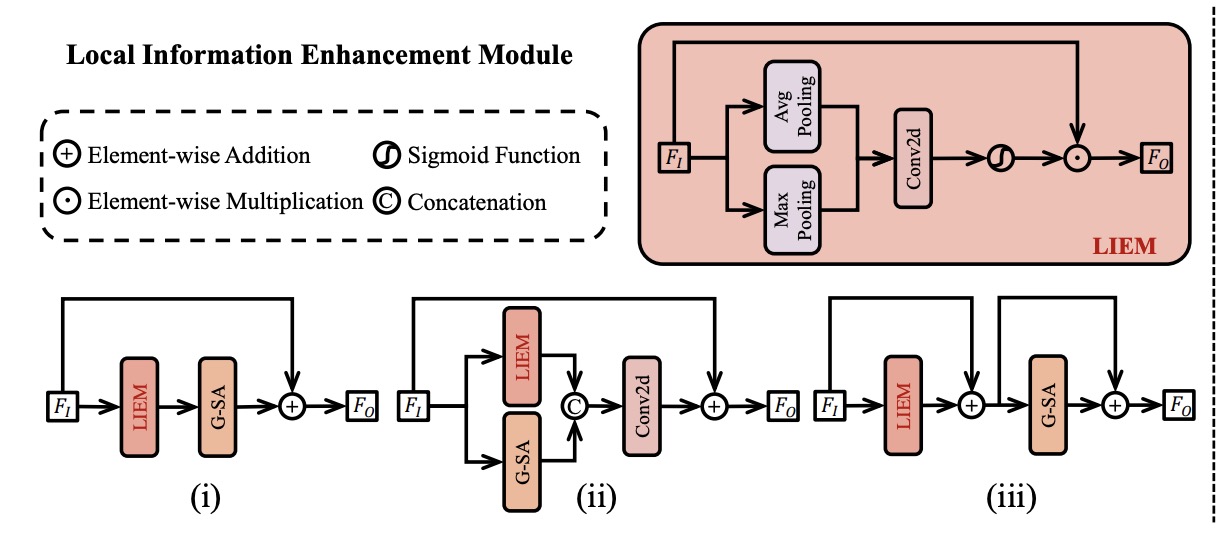
LIEM is introduced in order to address these shortcomings. Placed before the global attention block, it focuses on local details by combining average and max pooling to highlight key features, followed by processing with a global attention block.
Dynamic Frequency Loss
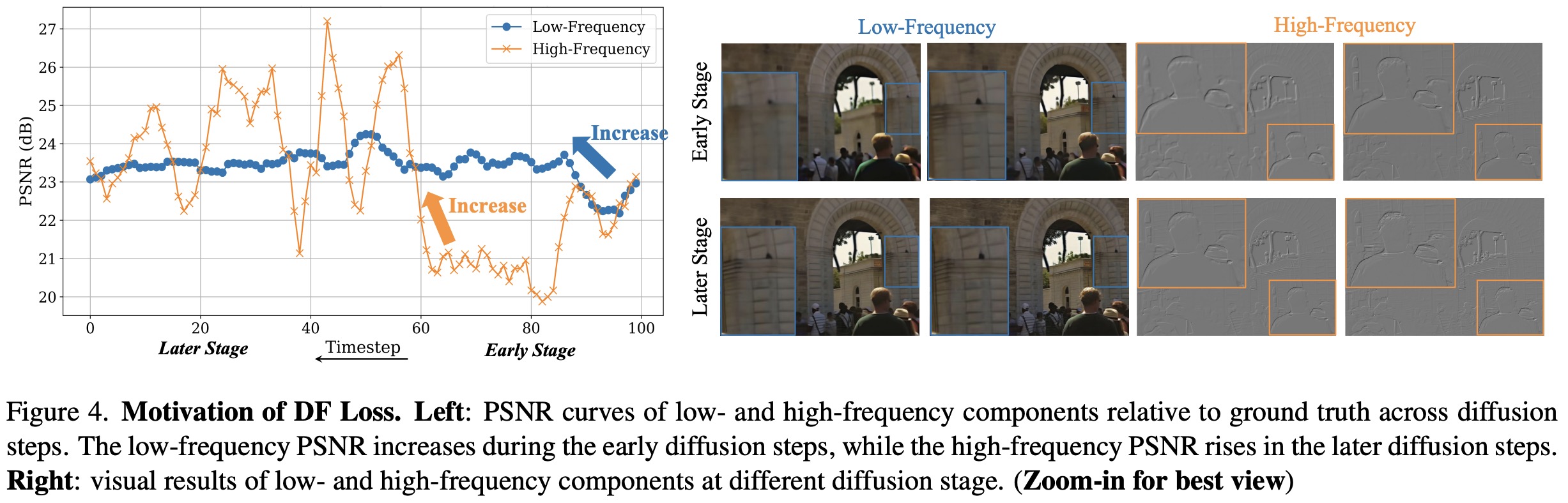
Diffusion models’ strong generative capacity can compromise fidelity in video restoration. Usually, during diffusion, early stages focus on reconstructing low-frequency structures, while later stages refine high-frequency details like edges and textures. This pattern suggests a need for a loss function that leverages this characteristic to optimize fidelity effectively.
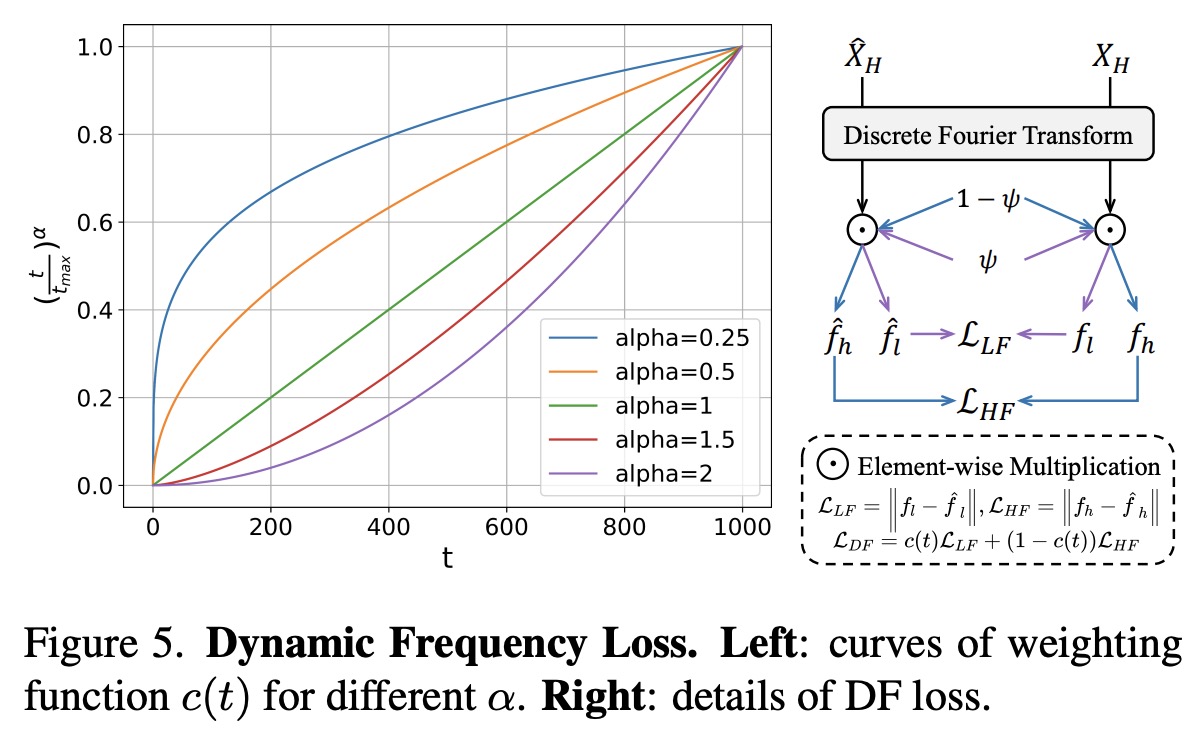
The proposed DF Loss addresses this by decoupling fidelity into low-frequency (structures) and high-frequency (details). At each diffusion step, the model reconstructs the latent video, applies a Discrete Fourier Transform to separate low- and high-frequency components, and calculates losses for both. A weighting function dynamically adjusts focus, prioritizing low-frequency fidelity in early steps and high-frequency fidelity later.
Experiments
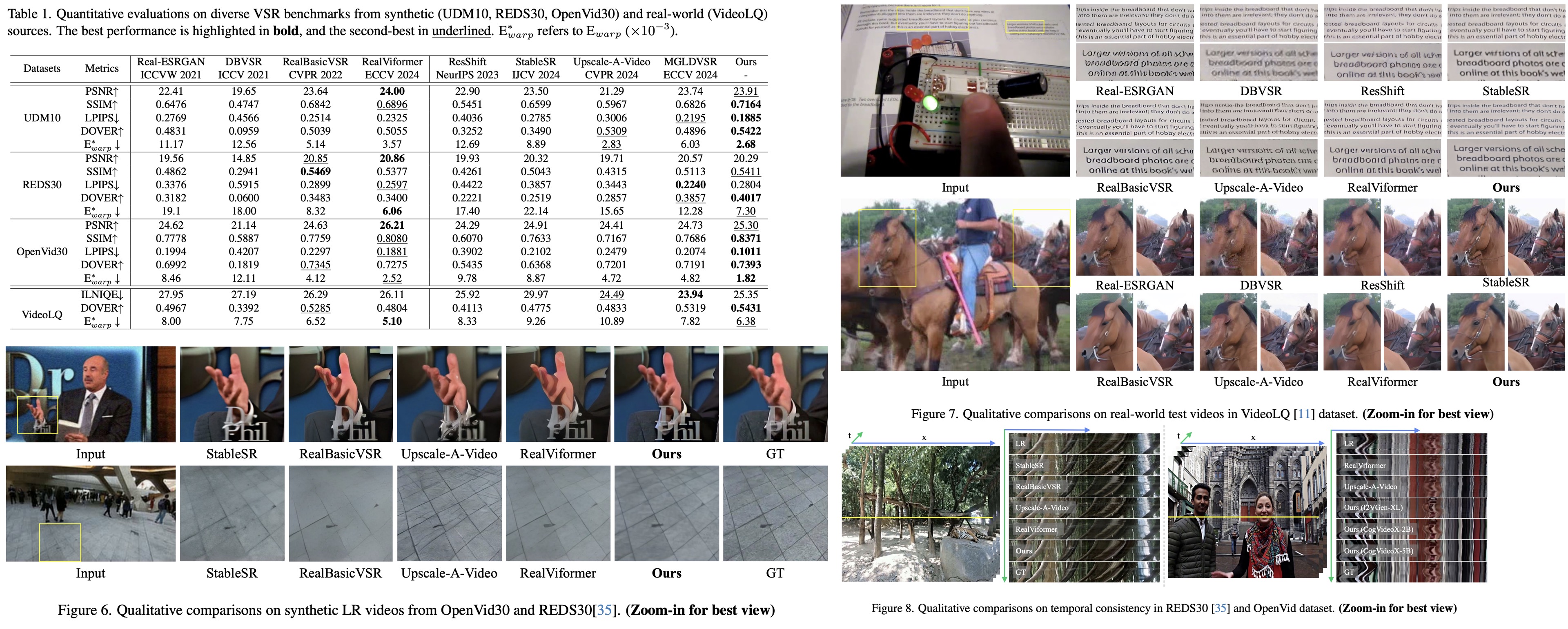
STAR is evaluated against state-of-the-art methods like Real-ESRGAN, DBVSR, RealBasicVSR, RealViformer, and others on synthetic and real-world datasets. It demonstrates superior performance in both quantitative and qualitative metrics:
- Quantitative Results: On synthetic datasets, STAR achieves top scores in four out of five metrics and second-best in PSNR, highlighting its ability to deliver realistic details, fidelity, and temporal consistency. On real-world datasets, STAR shows robust spatial and temporal quality.
- Qualitative Results: STAR generates the most realistic spatial details and effectively removes degradation artifacts. Examples show it excels in reconstructing fine structures like text, human hands, and animal fur, benefiting from the T2V model’s spatial and temporal priors and the DF Loss’s fidelity enhancements.
- Temporal Consistency: Compared to methods like StableSR and RealBasicVSR, which struggle with temporal consistency due to limitations like optical flow inaccuracies, STAR achieves the best results by leveraging the T2V model’s powerful temporal prior, eliminating the need for optical flow maps.